How to bring wildfires under control
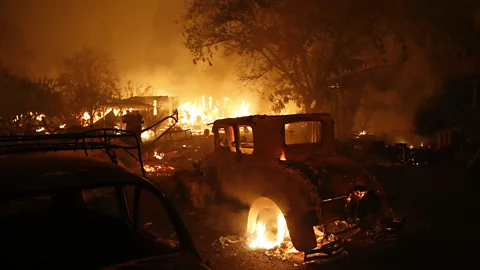
Earthquakes, tsunamis, flooding and wildfires are nature at their most fierce and uncontrollable. But predicting when and where they might strike could help save lives.
It’s the dead of the night in California, but the skies are alight. A crackling, searing heat spreads through the forest, destroying everything that crosses its path. With the lack of humidity, soaring winds, and an uninterrupted source of fuel, the fire spreads much faster than the authorities can handle.
This horror story unfolded last year when California saw its deadliest wildfires on record. A total of 8,527 fires burned in an area spanning 1,893,913 acres. In Paradise, California, 86 lives were lost when fire swept through their town in November last year.
But this was by no means limited to one state, or even one country. In 2018, fires broke out on the Greek coast, in the Australian bush, in the UK – and even in the Arctic Circle. It’s a worldwide problem.
You might also like:
• The signs of disease no one can see
These fires are linked to symptoms of climate change: droughts, increasing temperatures, shifting wind patterns and low humidity. With this in mind, we can expect more wildfires in the future.
But help might be coming from an unusual place.
Controlling the spread of forest fires requires a detailed understanding of the forests and how to manage them – something that is beyond all but a handful of specialised experts. This is where artificial intelligence steps in.
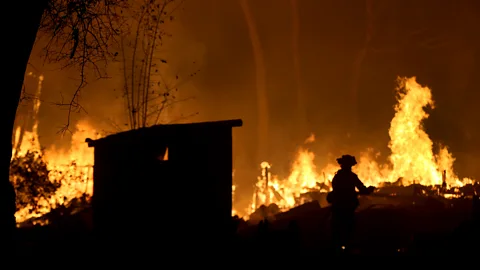
SilviaTerra is a company based in San Francisco using artificial intelligence to map forests, providing resources to help planners reduce the risk of fires.
“We combine on-the-ground measurements made in the forest with a large stack of remote sensing information and topographical data,” says Nan Pond, SilviaTerra’s lead biometrician. Her team uses satellite data along with aerial photographs and laser scanning techniques to measure the spread of vegetation. “[We] use our algorithmic process to produce high-resolution estimates of the sizes and species of trees present across large areas.”
Currently the team is mapping every forest in the US, 305.5 million hectares, to a resolution of 15 square metres. Once the maps are complete, machine learning algorithms will help authorities to identify the areas most at risk of fire.
The risk is evaluated by taking into account the concentration, species and the size of the trees in each area. Some trees, like pines, are much more flammable than others, such as maple. The difference comes down to many factors including how much oil they hold in their bark, the shape of the foliage and density of the leaves.
Previously, forest inventories involved taking random samples of a forest and mapping them on the ground, then scaling this up to the whole forest. Now, with SilviaTerra’s work, forest inventories are becoming much more accurate.
The firm has analysed the area around Paradise in California, for example, to create a map which shows areas of higher and lower wildfire risk. The most at-risk areas, identified in red on the map, are the places where authorities should be intervening in advance by putting in physical fire barriers or cutting trees down.
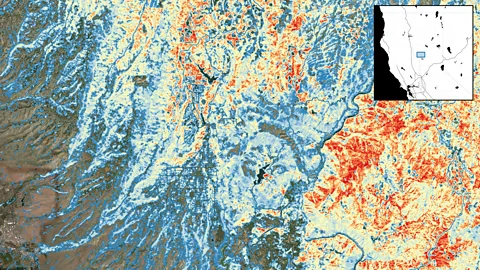
But it’s a complicated task. The algorithms have to juggle many conflicting priorities.
“Forest managers are working to mitigate the impacts of forest fire while balancing many other resources we get from forests,” says Pond. Forests are home to a wide variety of animal species; they’re also sources of clean water, used for recreational activities, help reduce erosion and suck up carbon from the atmosphere.
Fire is not the only natural disaster that could be tackled with this sort of approach. Flooding is also influenced by an unlucky confluence of extremely heavy rainfall, land use, drainage and the capacity of existing water courses. While most flood prediction models attempt to capture all these influences to provide flood warnings, they often give a fairly crude picture. Michael Souffront at Utah’s Brigham Young University is working to improve the Global Flood Awareness System by using AI to introduce smaller rivers and tributaries, which are currently excluded, into the models.
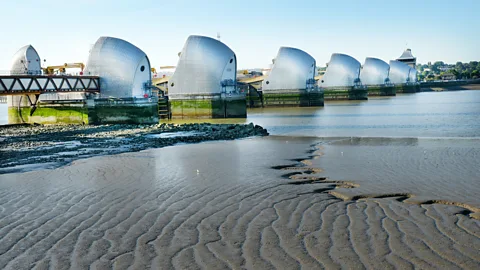
Smaller streams and waterways are vital for preparing for floods, as they are often where overflowing begins. Souffront also has created a web-based application that shows animated flood warnings on individual streams over time. This provides a level of detail not previously available to local governments, in close to real time. This far more detailed picture can then be put to use by those seeking to defend towns and cities from the risk of flooding.
In December 2015, the city of Leeds in the north of England was hit by some of the largest flood levels ever recorded. In response, the construction company Bam Nuttall was contracted to build flood defences. They employed technology start-up SenSat to create a virtual replica of the flood corridor, along a 12km length of the river Aire.
“The virtual flood corridor had one objective, to allow computers to analyse the river, topography and flood risks across vastly more variables than humans alone could do,” says James Dean, chief executive of SenSat.
Drones were flown along the river valley 80 metres above the ground, gathering pictures and taking a measurement every 2.5cm. In total it collected more than 600 million data points that were used to digitally reconstruct the river and its flood plain. Then AI was used to interpret the data.
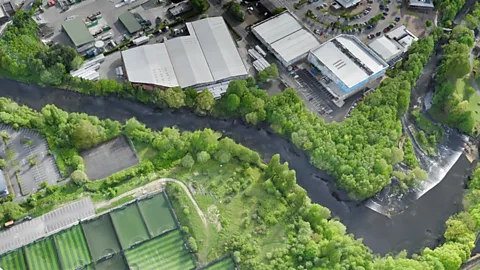
“In order to do this, we built something called an elastic spatial indexing algorithm that allows an AI to identify objects as individual items within a 3D reconstructed environment,” says Dean. “This produces better decision making and more efficient use of public funds to protect vulnerable areas and alleviate the misery of flooding.”
The resulting map helped inform what construction works Bam Nuttall needed to undertake to reduce flooding in the area. The building work is due to be completed by summer this year.
But there are some natural events that cannot be avoided. Earthquakes, volcanic eruptions and tsunamis are notoriously unpredictable and impossible to control. While these disasters often claim many lives immediately, what happens in the aftermath and how rescue teams react has a large impact on how many people survive.
A team at Tohoku University in Sendai, Japan is attempting to increase the chances of finding people and pulling them alive from the chaos that follows tsunamis, volcanoes and earthquakes.
In March 2011, Japan was hit by one of the most powerful earthquakes ever recorded in the region. It was the fourth most powerful earthquake on record, moving the entire island of Honshu by 2.4 metres and shifting the whole planet’s axis by around 17cm. The earthquake, which had its epicentre 130km off shore, triggered a tsunami that devastated a large part of the country’s east coast and triggered the now infamous emergency at the Fukushima Daiichi nuclear power plant. The Tohoku region was one of the most badly damaged. In total, the tsunami claimed nearly 20,000 lives. To this day 2,000 people are missing.
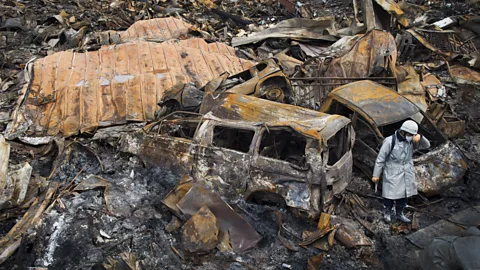
Seven years earlier, a tsunami hit 14 Asian and African countries after an earthquake in the Indian Ocean. An estimated 230,000 people died, and the damage was thought to be made worse by a lack of communication. The tsunami hit Indonesia first, then Thailand, Myanmar and Sri Lanka. Hours later, the tsunami hit the east African coast. Those in its path had little to no notice of what was coming because of a lack of early warning systems at the time. Rescue workers also struggled to find the places that needed their help most as communication networks had been decimated and whole regions were cut off.
Bai Yanbing at Tohoku University hopes his work can avoid these problems in the future. He is developing a tool that uses AI to define areas affected by natural disasters, classify the damage on the ground and alert governments and rescue teams to where they are most needed.
They do this by running satellite imagery captured in the immediate aftermath of a natural disaster through a machine learning algorithm that’s been trained to classify buildings into different categories of destruction and stability. It can identify buildings that have been totally destroyed, half damaged but reparable, slightly damaged or undamaged.
“This information can then be sent to first responders and give them the real-time information they need to save lives, and be safe themselves, when entering a post-disaster area” says Lucas Joppa, chief environmental officer at Microsoft, which has provided funding for Yanbing’s project under its AI for Earth programme. The system can tell rescuers where the worst affected areas are and where they should focus their efforts to find survivors.
Machine learning technology could not just help to coordinate the response to disasters, but also help in the rescue efforts themselves.
In these cases, robots may be the answer. Katia Sycara, director of the advanced-agent robotics laboratory at Carnegie Mellon University in Pittsburgh, is developing swarms of robots that will be able to go into disaster zones to autonomously search for survivors. The robots would use AI-powered machine vision to help them interpret what they are seeing and make their own decisions.
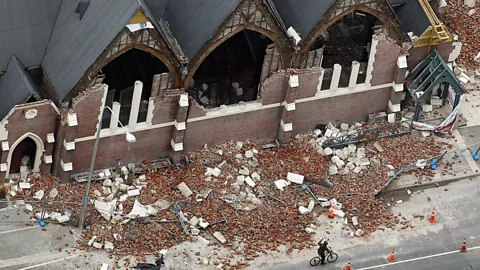
“This enables robots to go to areas that may be inaccessible and dangerous for humans and search for victims” says Sycara. The meltdown at the Fukushima nuclear power plant is one example where rapidly rising radiation levels made it unsafe for human disaster workers to go into the area.
The biggest challenge with these kinds of robots, says Sycara, is enabling them to move around in environments where the terrain is unknown and unexpected obstacles littering their path.
There are some researchers, however, who believe that artificial intelligence could also help provide an early warning of natural disasters themselves.
It is notoriously difficult to predict disasters like earthquakes and volcanoes. Researchers are edging closer to being able to predict aftershocks, however. Aftershocks can be just as devastating as the initial earthquake. In Christchurch, New Zealand, a magnitude 7.1 earthquake in September 2010 caused widespread damage but did not cause any deaths, yet a smaller aftershock that occurred five months later killed 185 people.
In a study last year, a team from Harvard University fed data from hundreds of thousands of earthquakes, including Japan’s 2011 disaster, into a neural network. The AI predicted the likelihood an aftershock would hit the surrounding area, which was divided up into 5km by 5km squares. By taking each square as its own problem, the AI predicted aftershocks more successfully than previous methods.
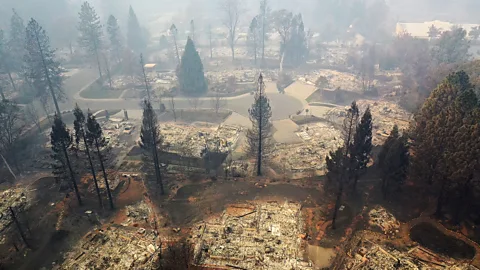
But when it comes to prediction of and preparation for natural disasters, one of the biggest barriers is having enough accurate data. These events are still extremely rare, so the quantities of data needed to train machine learning algorithms is thin on the ground. Satellite and aerial surveys are often not a priority when governments are responding to a crisis.
Natural disasters also involve large numbers of variables. A wide range of events can turn a small tornado into one with a magnitude of EF5 – where winds can exceed 200mph and are powerful enough to lift cars off the ground – or lead a volcanic eruption to trigger a tsunami, for example.
“The good news, though, and the reason we’re focused on this work, is that even incremental progress can [have a] exponential impact,” says Joppa. “A few more hours to evacuate an area, to adjust where resources are deployed to prevent flooding – those hours and minutes are often the difference between life and death.”
--
If you liked this story, sign up for the weekly bbc.com features newsletter, called “If You Only Read 6 Things This Week”. A handpicked selection of stories from BBC Future, Culture, Capital, and Travel, delivered to your inbox every Friday.